When Machines Beat Bias: What Algorithmic Trading Teaches Us About Rationality
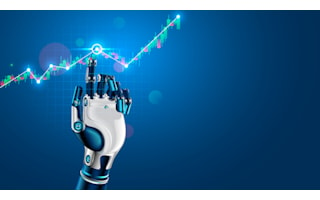
The debate over rationality in economics has long revolved around how closely human behavior matches the predictions of rational economic models such as expected utility theory (EUT). With the rise of algorithmic decision-making, the discussion has taken on new urgency. Do machines — often assumed to be cold, calculating, and consistent — actually make more rational decisions than humans or do they inherit biases from human programmers and training data?
In recent research, I set out to tackle these questions using detailed transaction-level data from the NASDAQ Copenhagen Stock Exchange, covering the period 2016–2017. This dataset includes over 100 million trades and uniquely distinguishes between trades executed by human traders and those executed by fully automated algorithmic traders (ATs) operating without human involvement.
The focus was on one of the most studied behavioral biases in finance: the disposition effect — the tendency to sell winning positions too quickly and hold onto losing positions too long. This effect has been documented across asset classes and investor types and is often explained by a combination of loss aversion, dependence on reference points, overconfidence, and mood.
What do the data show?
Human traders display a robust disposition effect, consistently realizing a significantly higher proportion of gains than losses. In contrast, ATs as a group, on average, realize gains and losses at nearly identical rates. This holds true across different algorithm types, including market-making and order execution systems, and across different matching methods controlling for trading frequency, turnover, and portfolio size.
When we drill down into the minority of algorithms that do show a positive disposition effect, the pattern is driven not by bias but by profitable contrarian trading strategies — deliberately buying declining stocks and selling rising ones. In contrast, the disposition effect among human traders cannot be fully explained by rational factors; rather, it appears at least partially rooted in psychology, as shown by its sensitivity to external influences like weather. This suggests that while human traders’ disposition effect reflects, at least in part, underlying psychological biases, similar patterns observed among algorithms are the result of deliberate, rational behavior.
Visual evidence: how trading behavior unfolds during the day
A particularly revealing picture comes from examining how the proportion of gains realized (PGR) and proportion of losses realized (PLR) evolve throughout the trading day. The figure below plots these measures from 10 am to 5 pm, separately for algorithms and humans, using carefully matched daily data.
Several insights emerge:
- Algorithms (red lines) show almost no gap between PGR (dashed red) and PLR (solid red). Both measures increase steadily and remain converged over the course of the day. This suggests that algorithms are largely insensitive to whether they are realizing gains or losses — a key hallmark of rational trading behavior.
- Humans (blue lines) display a persistent gap between PGR (dashed blue) and PLR (solid blue) across the entire day. They consistently realize a higher proportion of gains than losses, an unmistakable signature of the disposition effect.
- All four lines rise sharply near the end of the day, reflecting increased trading activity as the market approaches close — a pattern common to both humans and machines.
This simple graph vividly illustrates a key difference: whereas humans are swayed by the emotional pull of gains and losses, algorithms focus on execution without attachment.
Do external factors like weather matter?
A novel part of this research involved merging trading data with hourly weather data for the cities where traders were based. This allowed me to examine how exogenous mood-shifting factors — particularly morning temperatures — affect trading behavior.
The results show that human traders’ disposition effect is significantly stronger on colder mornings, consistent with psychological research linking lower temperatures to lower mood and impaired cognition. For algorithms, however, weather has no measurable effect — highlighting again their immunity to environmental influences that affect human judgment.
What does this mean for economics and markets?
These findings carry several important implications.
First, they provide field evidence that behavioral biases like the disposition effect are psychological in nature and that programmers, on average, appear disciplined enough to avoid hardwiring these biases into trading algorithms or adjusting parameters impulsively during live trading. As a result, algorithms tend to align more closely with rational economic models such as expected utility theory (EUT). Yet, it is important to note that this study primarily examines algorithms based on fixed rules. If future generations of algorithms — particularly those using machine learning — begin to inherit or amplify biases from training data, this could introduce new forms of irrationality into markets.
Second, the growing prevalence of rational algorithmic trading could enhance the ability of rational models to explain and predict real-world phenomena, including financial markets. If a larger share of market activity is carried out by agents free of cognitive biases, theoretical models grounded in rationality may perform better in practice.
Third, the fact that some algorithms successfully exploit contrarian trading strategies underscores a deeper point about market efficiency. While behavioral biases such as overreaction to the news create inefficiencies, they also generate opportunities for rational agents, human or machine, to profit from stock price mean reversion. This coexistence of behavioral patterns and rational arbitrage may be a defining feature of modern markets.
Looking ahead
The rise of automation is reshaping financial markets, but its impact on decision-making and overall market quality is still unfolding. While research shows that algorithmic traders — especially high-frequency traders — may improve market quality by enhancing liquidity and price efficiency through market-making and arbitrage, episodes like the 2010 “flash crash” demonstrate that algorithms can also contribute to sudden spikes in volatility. The evidence from this study that algorithms help traders overcome psychological biases suggests that algorithms may, in turn, contribute to more stable and efficient markets.
In short, algorithms don’t just speed up trading — they help financial markets come closer to the rationality that economic models have long assumed. As automation spreads, understanding when machines outperform humans, and why, will become increasingly important for researchers, regulators, and market participants alike.
0 Kommentarer